How to Use Artificial Intelligence to Improve Your Forex Market Trades
- Hammet Forex
- Dec 19, 2024
- 6 min read
1. Introduction to AI in Trading
Artificial Intelligence (AI) refers to the ability of machines to perform tasks that typically require human intelligence, such as learning, reasoning, problem-solving, and pattern recognition. In trading, AI applications range from predictive analytics to automated trading systems, all of which leverage machine learning (ML) algorithms to analyze market data and make decisions.
AI in trading involves using algorithms and data-driven strategies to predict market trends, execute trades, and manage risk. These systems can process huge datasets in real time, making decisions faster and more efficiently than human traders. By incorporating AI into trading strategies, traders can gain a competitive edge in highly volatile and fast-paced markets.
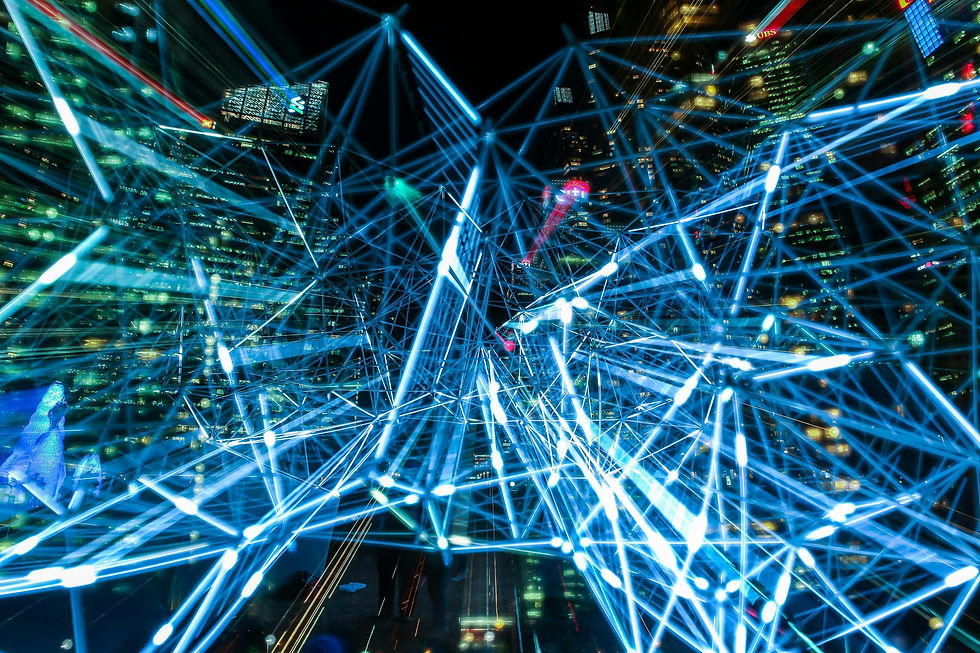
2. How AI Enhances Trading Strategies
AI-powered tools enhance trading strategies in several ways. Some of the key areas where AI can provide value include:
A. Predictive Analytics
One of the primary uses of AI in trading is predictive analytics. Machine learning algorithms can analyze historical market data to identify patterns, trends, and anomalies that are not immediately apparent to human traders. By recognizing these patterns, AI can forecast future price movements, allowing traders to make more informed decisions.
For instance, AI-based trading systems can predict stock price trends, currency fluctuations, and commodity movements. These predictions can guide traders in executing trades at the most opportune moments, maximizing profit and minimizing losses.
B. Automated Trading
AI enables automated trading, also known as algorithmic trading, where AI algorithms automatically execute buy and sell orders based on predefined criteria. These systems use real-time market data and algorithms to determine the optimal timing for a trade, eliminating emotional decision-making and human error.
Automated trading systems can execute high-frequency trades, which would be impossible for human traders to perform manually. This capability allows traders to take advantage of small price movements, increasing profitability over time.
C. Risk Management
Risk management is a critical aspect of trading, and AI can play a significant role in managing risk. AI-powered systems can analyze market conditions and assess the potential risks associated with a trade. These systems can adjust trading strategies in real-time to mitigate risks based on market volatility, news events, and economic data.
AI algorithms can also optimize the allocation of capital by diversifying portfolios and using risk models to minimize losses during adverse market conditions. By continuously learning from past performance, AI can refine risk management strategies, reducing the likelihood of significant drawdowns.
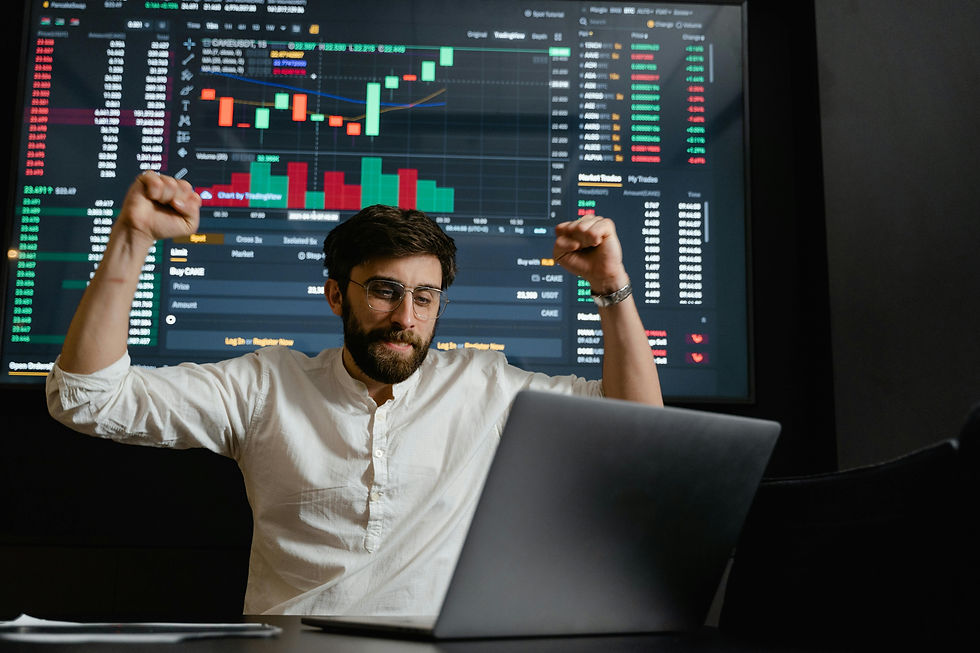
D. Sentiment Analysis
AI can analyze market sentiment through natural language processing (NLP) and machine learning. By processing news articles, social media, financial reports, and other textual data sources, AI systems can assess the overall mood of the market. This is particularly useful for identifying shifts in sentiment that could influence market movements.
For example, AI algorithms can detect trends in social media discussions about a particular stock or asset. If there is a surge in positive sentiment, it may indicate a potential buying opportunity, while negative sentiment could signal the need for caution. Sentiment analysis helps traders make more informed decisions based on real-time market sentiment.
3. Key Benefits of AI in Trading
There are numerous benefits to using AI in trading. Some of the most notable advantages include:
A. Speed and Efficiency
AI can process and analyze vast amounts of data in a fraction of a second. This enables AI-based trading systems to make decisions much faster than human traders, which is especially important in high-frequency trading (HFT) and day trading. With the ability to execute trades in milliseconds, AI systems can capture opportunities before they disappear.
B. Data-Driven Decision Making
AI removes emotions from trading by relying on data-driven decision-making. Traditional traders can be influenced by emotions such as fear, greed, and impatience, which often lead to poor trading decisions. AI, on the other hand, operates based on algorithms and data, ensuring that every decision is rooted in market facts and trends rather than emotional impulses.
C. Backtesting and Strategy Optimization
AI can backtest trading strategies using historical data, allowing traders to evaluate the effectiveness of a particular strategy before deploying it in live markets. This process involves running a strategy against historical market data to identify potential flaws and optimize the strategy for better performance.
AI systems can automatically optimize trading strategies based on historical performance and market conditions. By continuously refining strategies, traders can improve profitability and reduce risk over time.
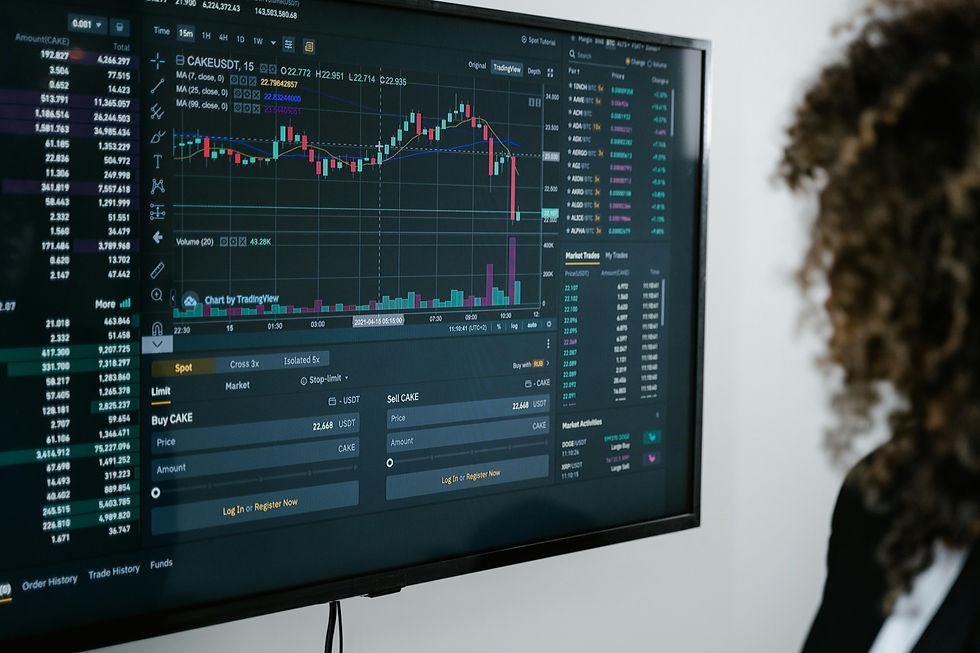
D. Reduced Human Error
Human traders are prone to errors, whether due to fatigue, emotional reactions, or simple mistakes. AI eliminates these factors, ensuring that trades are executed accurately and without bias. This reliability is especially important in fast-moving markets where timing is crucial.
E. Cost Efficiency
AI-powered trading platforms can reduce the need for human intervention, leading to cost savings for traders and investment firms. By automating repetitive tasks such as data analysis, trade execution, and risk management, AI can lower operational costs and increase profitability.
4. Types of AI in Trading
There are various types of AI applications in trading, each serving a specific purpose:
A. Machine Learning (ML)
Machine learning, a subset of AI, is widely used in trading to identify patterns, make predictions, and optimize trading strategies. ML algorithms can process large datasets, learn from past data, and make predictions based on statistical models. Over time, these algorithms improve their accuracy as they are exposed to more data.
Types of machine learning used in trading include:
Supervised learning: The algorithm is trained using labeled historical data to predict future market movements.
Unsupervised learning: The algorithm finds patterns in unlabeled data, which can be used to detect market trends or anomalies.
Reinforcement learning: The algorithm learns by interacting with the market environment, adjusting its actions to maximize profits and minimize losses.
B. Natural Language Processing (NLP)
NLP allows AI systems to process and analyze text data from various sources, such as news articles, financial reports, and social media. This enables AI to assess market sentiment and gauge the potential impact of news events on asset prices.
By using NLP algorithms, traders can stay ahead of breaking news, economic announcements, and social media trends, providing valuable insights that influence trading decisions.
C. Deep Learning
Deep learning, a subset of machine learning, involves training artificial neural networks to recognize complex patterns in data. This technique is particularly useful for processing large volumes of unstructured data, such as images, videos, and text. Deep learning models can be used for advanced predictive analytics, making them a powerful tool for forecasting market trends.
5. Challenges and Considerations When Using AI in Trading
While AI offers numerous advantages, it is not without challenges. Here are some considerations when implementing AI in trading:
A. Data Quality and Availability
AI systems rely heavily on high-quality data for accurate predictions. If the data fed into an AI algorithm is incomplete, inaccurate, or biased, it can lead to suboptimal trading decisions. Therefore, it is essential to ensure that the data used is reliable, clean, and up-to-date.
B. Overfitting
One of the risks in machine learning is overfitting, where an algorithm becomes too tailored to historical data and fails to generalize to new market conditions. This can lead to poor performance in real-world trading scenarios. To mitigate this, it is important to regularly retrain models using fresh data and ensure they are robust enough to handle changing market dynamics.
C. Regulatory Compliance
The use of AI in trading raises concerns regarding regulatory compliance. Financial authorities are closely monitoring the adoption of AI in trading to ensure that it adheres to market regulations, such as those related to market manipulation, insider trading, and transparency. Traders and firms using AI must stay informed about the regulatory landscape and ensure that their AI systems are compliant with applicable laws.
D. Ethical Considerations
AI-based trading systems can lead to ethical dilemmas, such as market manipulation or unfair advantages for certain market participants. As AI continues to evolve, it is crucial to ensure that AI-driven trading is conducted in an ethical and responsible manner.
6. Conclusion
AI is transforming the world of trading, offering significant advantages to traders who adopt it effectively. By leveraging AI for predictive analytics, automated trading, risk management, and sentiment analysis, traders can make more informed decisions, increase efficiency, and boost profitability. While challenges such as data quality, overfitting, and regulatory compliance must be addressed, the potential rewards of incorporating AI into trading strategies are substantial.
AI in trading
Artificial intelligence for trading
AI trading strategies
Machine learning in finance
Predictive analytics in trading
Automated trading systems
AI for risk management
Sentiment analysis in trading
Benefits of AI in trading
Algorithmic trading with AI
High-frequency trading with AI
Trading with machine learning
Comments